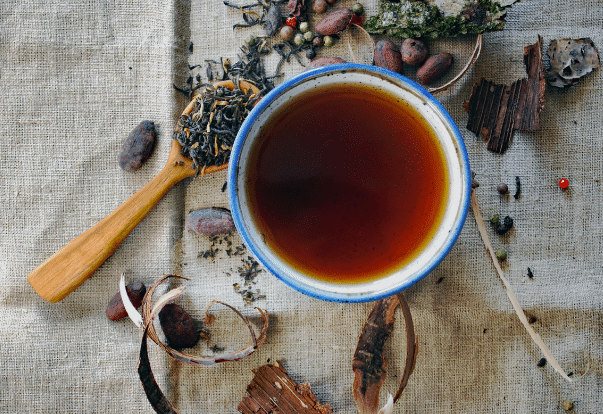
In the middle ages, “reading the tea leaves” was the go-to method for predicting the future. Here is how it worked. The fortune-teller would have the subject drink unstrained tea. When the drink was finished, the remaining residue in the bottom of the cup was analyzed for symbols or messages. Seeing a wild beast foretold misfortune. A bird meant good luck was coming your way. Ants indicated an ominous or evil omen, while angels suggested positive developments in your love life. How about a giant squirrel riding on the back of a flying unicorn? Well, you probably drank something other than tea.
Every day, across your entire organization, there is uncertainty. Choosing the right path in business always involves some guesswork. The future is never perfectly clear. But despite this uncertainty, your business moves forward. Decisions are made. Some end up being good decisions, while others do not generate the outcomes that were anticipated. But what if you could reduce the level of uncertainty, thus improving the overall quality and accuracy of these decisions? Even a small, incremental improvement in decision-making throughout your business can drive significant bottom-line results.
The first step toward reducing uncertainty in your business is leveraging sound analytical practices across your complete data landscape. Improved access to the right data by the right people at the right time, coupled with insightful analysis and data visualization, will lead to better decisions and better results. For this reason, most organizations are putting data at the center of their digital transformation strategy. Armed with deeper and more timely insights, decision-makers at all levels of the organization can more easily identify new opportunities to increase revenues and lower costs.
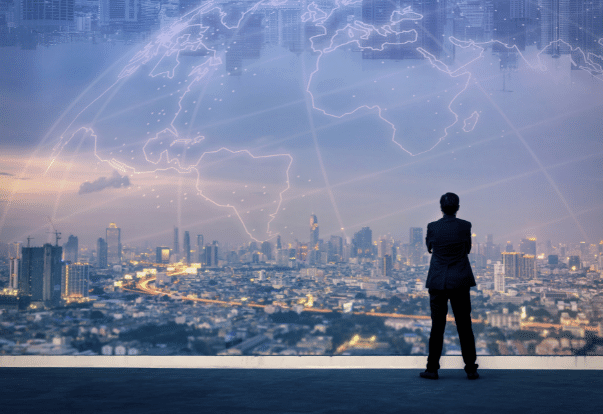
Regardless of where your organization is in its journey, digital transformation is a large and complex challenge. As with any challenge, the important thing is to get started. Take that first step … tackle the first problem … and then move on to solving the next one. So, how does your organization become more data-driven in its decision-making? Start by establishing a solid foundation of basic analytical skills. Training your team, at all levels and functions, on how to use data correctly is vital to reducing uncertainty and improving the quality of your decisions.
Emylla is ready to help you take that first step –- for free! Contact Emylla to schedule a complimentary webinar program, customized to your organization, covering the basics of statistical analysis. We will conduct as many sessions as you need, delivered in a light, concise, and easy to understand manner (no tea leaves though!). As a preview, included below is a basic review of a few statistical measures, their Microsoft Excel functions, and situations where they apply in business.
# # #
Describing and Summarizing Data
Range: The difference between the largest (maximum) and smallest (minimum) value.
Excel Fx: =MAX() – MIN()
Example: Data set {3,3,8,9,16,93}. The range here is 90 (93-3). A quick look at the range of your data can help you identify possible outliers, such as 93 in this data set.
Mode: The value in your data set that appears most often.
Excel Fx: =MODE()
Example: Data set {3,3,8,9,16,93}. The mode is 3 because it appears twice and all other values just once. Mode, for example, can be used to analyze categorical or list data, such as survey responses and product quality metrics (i.e. pass, fail, or hold).
Mean: The arithmetic average. The sum of all values divided by the number of values.
Excel Fx: =AVERAGE()
Example: Data set {3,3,8,9,16,93}. Add up all the values (132) and divide by the number of values (6) for a mean of 22. Mean, or average is typically used on data with a roughly normal distribution.
Median: The “middle” number – The mid-point of your data, where 50% of the data lies below the median value, and 50% above.
Excel Fx: =MEDIAN()
Example: Data set {3,3,8,9,16,93}. With an even number of data points, as in this example, you will have two values in the middle (i.e. 8 and 9). We get the median then by averaging the two middle numbers. In this example, the median is thus 8.5.
When our data is skewed, such as in the example data set, then the median serves as a better measure of “central tendency” compared to the mean. This is because the median is less sensitive to outliers. The mean (22) is heavily influenced by the outlier 93. The median (8.5), however, is not and thus provides a better measure of “the center” of this data set.
Understanding the Shape of Data
How is your data distributed? Summary statistics like mean, median, mode, and range need some context, particularly if you want to make a prediction based on the data. Understanding the volatility within your data is also important. Volatility is the amount that your data changes over time. Lower volatility datasets are more stable and lead to more reliable predictions. Higher volatility brings greater uncertainty. Here are a few tools to understand volatility, and the overall “shape of your data.”
Variance: A measure of how far your data lies from its mean.
Excel Fx: =VAR()
The variance is calculated by subtracting each value from the average for the entire data set and then squaring the difference. We square the value to get rid of any negatives (i.e. data points that are below the mean), otherwise, the positive and negative values would cancel each other out.
The variance of a large data set will be HUGE by nature since we have more values to square and sum. Since the variance is impacted by the number of values in the data set, it can make it difficult to use for comparison purposes. Standard deviation, which is the square root of the variance, provides a better (i.e. normalized) way to measure the spread of your data, while returning the units of measure back to the original form.
Standard Deviation: Measures how spread out your data is, relative to the mean.
Excel Fx: =STDEV()
Standard Deviation is calculated by taking the square root of the variance. A low standard deviation indicates that the values tend to be closer to the mean (i.e. not very spread out), while a high standard deviation indicates that the values dispersed farther from the mean. Standard deviation is used on data that is approximately normal, with a shape like a bell curve. The flatter the curve, the greater the spread (higher standard deviation). A steep curve indicates the data is more closely clustered around the mean (lower standard deviation).
Data Modeling Tools
After exploring a data set with some of the descriptive tools identified above, we can dig deeper to see if there is a relationship between variables in the data. What is the association in the movement of certain variables over time? Covariance and correlation can help us understand these relationships.
Covariance: With covariance, we are trying to determine if two variables tend to move together over time (i.e. strong relation), or if their movement is more random (i.e. weak relation). Covariance is calculated by first subtracting each variable’s data point from its mean (like variance described above), and then multiplying the two values together. We then add all the products together and divide by the number of samples (less one). But it is always easier just to use the built-in Excel function!
Excel Fx: =COVAR()
The covariance, like variance, is a huge number and is not normalized in any way. Therefore, it can be difficult to interpret. Generally, a large and positive covariance will indicate a strong relationship between the variables.
Correlation: To provide a normalized measure of the relationship between variables, we divide the covariance by the product of each variable’s standard deviation.
Excel Fx: =CORREL().
The resulting value, the correlation between the variables, will always be between -1 and 1. If the correlation is closer to -1, this indicates a strong negative correlation (i.e. when one goes up, the other goes down). A correlation closer to +1 indicates that the variables move together. A correlation closer to 0 means there is not a strong relationship between the variables.
A final word. Remember not to confuse correlation with causation. Just because two variables are correlated, does not mean there is a causal relationship. For example, ice cream sales and shark attacks are two strongly correlated data sets in the United States. However, no one would say that eating ice cream somehow causes increased shark attacks. The “missing” variable here is summer. In the summer months, more people tend to go to the beach and more people tend to go out for ice cream.
Contact us today to schedule the free distance learning program!
© Emylla LLC (June 2020)